Leading Ai Technology Companies
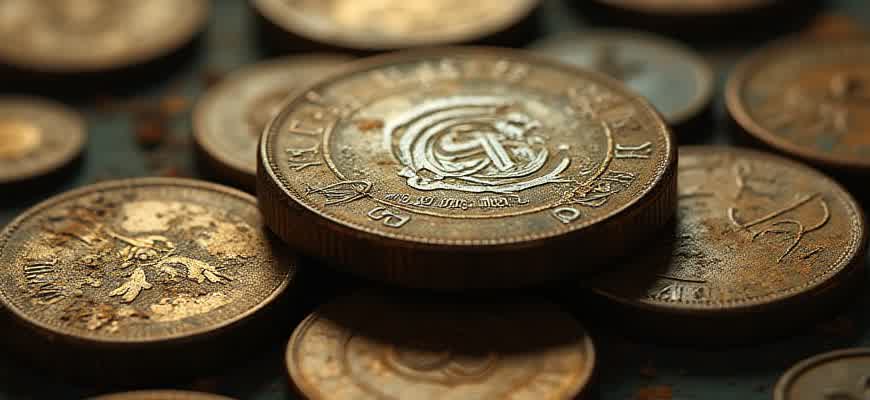
The intersection of artificial intelligence and cryptocurrency has become increasingly significant as AI-driven solutions are transforming how digital currencies are mined, traded, and managed. A few leading companies in AI technology are at the forefront of this evolution, developing sophisticated algorithms that improve the security, efficiency, and scalability of blockchain-based systems. These companies not only drive advancements in AI but also contribute to solving critical challenges in the crypto space, such as transaction speed and fraud prevention.
- OpenAI - Known for developing cutting-edge AI models, OpenAI's technologies are leveraged in smart contract execution and blockchain analytics.
- IBM - IBM's Watson AI platform is integrated into crypto systems to enhance predictive analytics and offer valuable insights into market trends.
- Google DeepMind - Pioneering reinforcement learning techniques, DeepMind collaborates with crypto projects to optimize trading algorithms.
AI has the potential to revolutionize the way cryptocurrencies function, from improving transaction verification to enhancing user privacy.
These companies apply machine learning and neural networks to solve complex problems within the crypto space. Whether through automating decision-making processes or improving blockchain protocols, the integration of AI offers a major leap forward in the performance and reliability of digital currencies.
Company | Key Contribution |
---|---|
OpenAI | AI-driven market prediction and smart contract optimization |
IBM | Blockchain security and transaction analysis |
Google DeepMind | AI-enhanced trading algorithms and blockchain scalability |
AI Tech Giants: Transforming the Landscape of Cryptocurrency
Artificial intelligence is rapidly reshaping the cryptocurrency industry. Leading AI companies are playing a pivotal role in enhancing blockchain security, improving transaction speeds, and facilitating smarter trading strategies. By applying machine learning models to market trends, AI has significantly transformed how cryptocurrencies are analyzed and traded globally.
Tech leaders in AI are not only focusing on automation but are also developing solutions that ensure scalability and efficiency for decentralized finance (DeFi) platforms. These innovations are addressing the challenges of high volatility and providing users with more predictive and actionable insights into crypto assets.
Key Innovations in AI for Cryptocurrency
- Blockchain Security: AI-driven solutions can detect suspicious activities or vulnerabilities in real-time, reducing the risk of hacks and fraud.
- Predictive Trading: AI algorithms analyze historical data to identify patterns and forecast price fluctuations, enabling more accurate investment decisions.
- Smart Contracts: AI-powered contracts can be self-executing with minimal human intervention, increasing efficiency and reducing errors.
Companies Leading the Charge
- OpenAI: Through its advanced machine learning models, OpenAI has contributed significantly to the automation of trading bots and predictive analysis within the crypto market.
- IBM Watson: Known for its AI capabilities, IBM is integrating blockchain solutions with its cognitive computing systems to streamline crypto transactions.
- Google DeepMind: DeepMind's AI systems are being utilized to enhance the scalability and security of blockchain technologies.
"AI's ability to learn from vast amounts of crypto market data is revolutionizing the way investors approach digital assets." – Industry Expert
AI-Driven Cryptocurrency Platforms
Platform | AI Application | Impact |
---|---|---|
HaasOnline | AI-powered trading bots | Improved automation and strategic execution for traders |
Endor Protocol | Predictive analytics based on blockchain data | Empowered users with more precise predictions for crypto market trends |
SingularityNET | Decentralized AI network | Integration of AI with blockchain for seamless DeFi solutions |
How AI Companies Are Revolutionizing Healthcare
Artificial intelligence is playing an increasingly significant role in the healthcare sector, transforming everything from diagnosis to patient care. Leading AI firms are developing advanced solutions that aim to enhance clinical workflows, improve treatment outcomes, and lower healthcare costs. By integrating deep learning algorithms and machine learning models, these companies are empowering healthcare providers to make faster and more accurate decisions based on vast amounts of medical data.
AI's influence on healthcare is particularly evident in areas such as drug discovery, personalized treatment plans, and diagnostic tools. Companies at the forefront are working on predictive models that can anticipate patient conditions before they become critical, and using AI to analyze medical images, genetic data, and electronic health records to detect diseases earlier and more precisely.
Key AI Innovations in Healthcare
- Predictive Analytics: AI systems are being used to analyze patient data and predict future health risks, enabling doctors to intervene earlier.
- Drug Development: AI accelerates the drug discovery process, analyzing compounds and predicting their efficacy before clinical trials.
- Personalized Medicine: Machine learning algorithms help create customized treatment plans by analyzing genetic data and medical histories.
AI-driven systems have the potential to reduce diagnostic errors and provide faster, more precise treatments, fundamentally transforming the landscape of modern healthcare.
Impact of AI on Healthcare Efficiency
AI's ability to automate repetitive tasks and optimize workflows is helping reduce the burden on healthcare professionals. AI-powered systems can handle administrative tasks such as patient scheduling, insurance verification, and billing, allowing healthcare providers to focus more on patient care. This shift not only enhances operational efficiency but also improves the overall quality of care.
AI Application | Impact on Healthcare |
---|---|
Predictive Analytics | Reduces hospital readmissions and improves patient outcomes. |
Medical Imaging | Enhances diagnostic accuracy and speeds up image interpretation. |
Robotic Surgery | Offers more precision and reduces recovery time for patients. |
Key Drivers of Innovation in AI-Powered Financial Solutions
In recent years, artificial intelligence has transformed the financial sector, offering a variety of new opportunities for improving efficiency, security, and accessibility in financial services. AI-driven technologies are rapidly becoming essential in areas such as risk assessment, fraud detection, and personalized banking services. With the rise of cryptocurrencies and blockchain technology, AI is playing an even greater role in managing financial data and automating complex tasks.
As financial institutions continue to embrace AI, there are several key factors propelling innovation in this space. By enhancing decision-making capabilities and streamlining processes, AI is helping companies adapt to a rapidly changing economic environment. Below are some critical elements contributing to the ongoing evolution of AI-powered financial solutions.
Factors Influencing AI Innovation in Financial Solutions
- Data Availability and Quality: AI systems require vast amounts of high-quality data to make accurate predictions. With the proliferation of blockchain and cryptocurrency data, AI models can now analyze financial trends in real-time.
- Advancements in Machine Learning: Machine learning algorithms are becoming more sophisticated, allowing AI to better understand market behaviors and predict financial outcomes.
- Security and Fraud Prevention: AI is increasingly used to detect irregular patterns in transactions, helping to combat fraud and security breaches in digital financial platforms.
- Regulatory Compliance: AI technologies assist financial institutions in maintaining compliance with ever-evolving regulatory requirements, automating reporting and audit processes.
AI in Cryptocurrency and Blockchain
Blockchain and cryptocurrency markets have further accelerated AI adoption in the financial industry. AI technologies can streamline cryptocurrency trading by analyzing patterns and predicting price movements. Additionally, blockchain’s decentralized nature provides AI systems with transparent, tamper-proof data for more effective decision-making.
"AI is essential for optimizing the operational efficiency of cryptocurrency exchanges by reducing manual error and speeding up transactions."
Impact on Financial Institutions
Application Area | AI Contribution |
---|---|
Fraud Detection | AI models analyze transaction patterns to identify suspicious activities and reduce fraud. |
Personalized Financial Advice | AI-driven chatbots and virtual assistants provide tailored investment and financial planning advice. |
Risk Management | AI algorithms assess market risks and assist in portfolio diversification to minimize financial losses. |
How AI Firms Are Shaping the Future of Retail Through Intelligent Automation
The intersection of AI technology and the retail industry has led to a transformation, particularly through the integration of smart automation. AI-driven automation tools are streamlining operational processes, enhancing customer experiences, and enabling retailers to scale faster. With the rise of cryptocurrency, AI has also become a pivotal player in enhancing financial transactions, improving the security of digital payments, and providing predictive analytics for better market forecasting. AI companies are not only focusing on customer-facing solutions but also behind-the-scenes technologies that optimize supply chains and manage inventory in real-time.
The role of artificial intelligence in retail automation extends beyond simple task execution. AI systems leverage machine learning and predictive analytics to anticipate consumer behavior, offering personalized recommendations and optimizing pricing strategies. Furthermore, through blockchain technology, transactions are becoming more secure and transparent, which boosts customer confidence in digital and cryptocurrency payments. Here are some of the ways AI is influencing retail automation:
- Personalized Shopping Experience: AI systems analyze browsing patterns and preferences to provide tailored product recommendations.
- Inventory Management: Machine learning algorithms track product demand, ensuring stock levels are optimized and reducing waste.
- Dynamic Pricing: AI adjusts product prices based on demand, competition, and consumer behavior in real-time.
- Fraud Prevention: AI utilizes blockchain to secure transactions, ensuring safer cryptocurrency payments.
“Artificial intelligence is not just enhancing retail efficiency, it’s revolutionizing the customer experience. Through predictive models, companies can now offer bespoke services that were once unimaginable.”
AI-driven automation tools in retail are reducing manual labor and increasing operational efficiency. The integration of cryptocurrency in AI systems has also opened new opportunities for cross-border payments, ensuring faster, cheaper, and more secure financial transactions. These advances are crucial for businesses looking to stay ahead in a rapidly evolving market.
- Enhanced Customer Insights through AI Analytics
- Blockchain Integration for Secure Payments
- Increased Operational Efficiency
- Real-Time Data for Better Decision Making
Technology | Application in Retail |
---|---|
AI Machine Learning | Personalization and Inventory Optimization |
Blockchain | Cryptocurrency Payments and Security |
Predictive Analytics | Demand Forecasting and Dynamic Pricing |
The Role of Leading AI Companies in Advancing Autonomous Vehicles
The involvement of artificial intelligence companies in the development of self-driving vehicles has become a pivotal factor in the automotive industry's transformation. These companies leverage advanced AI algorithms and machine learning models to enhance the perception, decision-making, and navigation capabilities of autonomous vehicles. By integrating real-time data processing and simulation tools, AI companies are accelerating the creation of safer and more efficient vehicles capable of operating without human intervention.
Major players in the field have contributed significantly to the progress of autonomous driving technologies. AI firms focus on key aspects like object detection, path planning, and vehicle-to-vehicle communication, helping create a roadmap for fully autonomous transportation. Below are some of the key areas where AI companies are actively making an impact.
Key Areas of Impact
- Real-Time Data Processing: AI firms use sophisticated algorithms to process vast amounts of data from sensors like LiDAR, cameras, and radar, enabling real-time decision-making and obstacle avoidance.
- Machine Learning Models: Continuous training of AI systems on extensive datasets improves the accuracy of vehicle behavior predictions, ensuring better responses to unpredictable road conditions.
- Vehicle-to-Vehicle Communication: Autonomous vehicles can share data with each other, improving coordination and reducing the likelihood of accidents through enhanced situational awareness.
AI Companies Leading the Way
- Tesla: Pioneering autonomous driving with its "Autopilot" system, Tesla integrates deep learning and neural networks for vehicle navigation and decision-making.
- Waymo: A subsidiary of Alphabet, Waymo uses AI to create fully autonomous vehicles, focusing on real-time data processing and advanced perception systems.
- Mobileye: Specializing in computer vision and machine learning, Mobileye offers technology for both partial and fully autonomous driving systems.
"The future of transportation will be driven by AI advancements, where autonomy and safety become the standard on our roads."
Technological Contributions Overview
Technology | Description |
---|---|
AI-Powered Vision Systems | Utilizes computer vision and deep learning to interpret road signs, traffic lights, and pedestrians in real-time. |
Predictive Algorithms | Forecasts vehicle and pedestrian movements to optimize navigation paths and avoid collisions. |
Advanced Simulation Tools | Simulates various driving scenarios to test vehicle responses before real-world implementation. |
AI and Cybersecurity: How Leading Companies Are Securing the Digital Landscape
As the rise of blockchain and cryptocurrencies continues to evolve, the demand for robust cybersecurity measures has never been higher. Cyber threats in the crypto world are becoming increasingly sophisticated, and companies are turning to artificial intelligence (AI) to protect their digital assets. AI’s role in cybersecurity goes beyond traditional methods, incorporating predictive analytics, real-time threat detection, and automated response systems to stay ahead of malicious actors.
Leading technology companies are integrating AI with cutting-edge security solutions to create a safer digital environment for cryptocurrency transactions. These systems help identify vulnerabilities before they can be exploited and enable quicker responses to emerging threats, all while minimizing human error in handling complex security breaches.
AI-Driven Security Innovations in Cryptocurrency
Several top-tier companies are deploying AI-powered tools to safeguard digital currencies and blockchain networks. Here are some key strategies being used:
- Predictive Threat Intelligence: AI algorithms are trained to analyze past cyberattack patterns and predict potential future threats, allowing for proactive defense mechanisms.
- Real-Time Fraud Detection: AI-based systems monitor transactions 24/7 to detect anomalies in real time, preventing unauthorized activities such as hacking or double-spending.
- Automated Incident Response: Using machine learning, AI can autonomously respond to detected threats, such as isolating compromised accounts or suspending suspicious transactions, thereby minimizing damage.
Key Players in AI-Driven Crypto Security
Leading companies in the AI and cybersecurity space are setting the standard for innovation in securing cryptocurrency operations:
- Chainalysis: Known for its blockchain analytics platform, Chainalysis uses AI to track and analyze cryptocurrency transactions, helping to identify illicit activities such as money laundering and fraud.
- CipherTrace: Specializing in cryptocurrency forensics, CipherTrace uses AI to enhance its ability to detect suspicious transactions and track cybercriminal behavior across multiple blockchains.
- Darktrace: With its AI-driven threat detection and response tools, Darktrace uses machine learning to monitor blockchain networks and automatically mitigate risks in real time.
Emerging Trends in Crypto Security
As AI continues to evolve, several emerging trends are shaping the future of cryptocurrency security:
Trend | Impact |
---|---|
AI-Powered Encryption | Enhanced encryption methods based on AI algorithms make it more difficult for hackers to decrypt sensitive data, ensuring higher security standards for wallets and exchanges. |
Behavioral Analytics | AI models analyze user behavior patterns to detect anomalies, allowing for quick identification of malicious actors or unauthorized activities within the crypto ecosystem. |
Blockchain Security Protocols | AI is being used to design more robust consensus algorithms, improving the overall security and scalability of blockchain networks. |
“The future of crypto security lies in AI’s ability to predict, detect, and neutralize threats in real time, ensuring a safer digital landscape for all participants.” – Industry Expert
The Influence of AI on Manufacturing: Enhancements in Automation and Production
The integration of artificial intelligence (AI) into manufacturing processes is reshaping industries by improving production efficiency and operational flexibility. AI-driven technologies enable businesses to automate complex tasks, enhance precision, and reduce human error. The resulting improvements are not just in speed but also in the quality of products being manufactured. By utilizing AI, companies can optimize workflows and reduce costs, creating a more sustainable and competitive manufacturing environment.
In addition to automation, AI plays a critical role in predictive maintenance, which reduces downtime and increases productivity. Smart systems are capable of analyzing equipment data in real time to forecast potential failures and schedule maintenance activities before breakdowns occur. This proactive approach to machinery management extends the life cycle of assets and enhances the overall reliability of manufacturing processes.
Key AI Applications in Manufacturing
- Automation of Repetitive Tasks: AI-driven robots and systems perform repetitive tasks more accurately and consistently than human workers.
- Quality Control: AI can detect defects during the production process, ensuring products meet quality standards.
- Supply Chain Optimization: AI algorithms predict demand fluctuations, optimizing inventory management and reducing waste.
Benefits of AI in Manufacturing
- Increased Productivity: Automation accelerates production timelines while minimizing errors.
- Cost Efficiency: AI helps reduce operational costs through better resource management and predictive maintenance.
- Customization: AI enables manufacturers to offer personalized products with shorter lead times.
"AI technology in manufacturing is not just a trend but a fundamental shift toward more intelligent and efficient production systems."
AI in Action: A Comparative Overview
Technology | Impact on Manufacturing |
---|---|
Machine Learning | Improves decision-making by analyzing production data and identifying optimization opportunities. |
Robotic Process Automation | Automates manual tasks, reducing labor costs and improving consistency in production. |
Predictive Analytics | Enables proactive equipment maintenance, reducing unexpected downtime and increasing productivity. |
Leveraging AI for Personalized Marketing: A Game-Changer for Advertising
Artificial Intelligence has revolutionized many industries, and advertising is no exception. By incorporating AI technologies, companies are now able to offer hyper-targeted and personalized experiences for their customers. In the context of cryptocurrency, where the market is highly dynamic and user preferences are diverse, the role of AI in delivering tailored marketing strategies has become increasingly essential.
AI allows cryptocurrency businesses to analyze large sets of consumer data, predict behavior, and create personalized ad campaigns that resonate more effectively with individual users. With such precision, marketers can engage their audiences in ways that were previously unimaginable, driving greater engagement and conversion rates.
How AI Transforms Advertising in the Crypto Industry
- Predictive Analytics: AI models can forecast consumer actions based on historical data, enabling crypto companies to design personalized offers before users even express interest.
- Enhanced Targeting: By analyzing patterns in social media, transaction behavior, and online browsing, AI enables advertisers to identify the best audience segments for crypto products.
- Dynamic Content Creation: AI-driven tools can automatically adapt the content of ads, adjusting the messaging to the preferences of each user.
"AI-powered advertising in the cryptocurrency industry allows businesses to tap into granular insights that drive targeted marketing strategies, optimizing ROI on campaigns."
Key Benefits for Cryptocurrency Marketing
- Increased Customer Engagement: AI allows for continuous optimization of campaigns, leading to higher engagement rates and improved customer loyalty.
- Cost Efficiency: AI reduces ad spend wastage by delivering the right message to the right audience at the right time.
- Real-Time Adjustments: AI algorithms can quickly react to shifts in user behavior, ensuring that marketing strategies remain relevant and effective.
AI Technology | Benefit |
---|---|
Machine Learning | Improves targeting precision by analyzing consumer data and predicting future behavior. |
Natural Language Processing | Enhances customer interaction through personalized, conversational experiences in ads. |
Deep Learning | Optimizes ad content by understanding complex patterns in consumer data. |