Contents of Artificial Intelligence
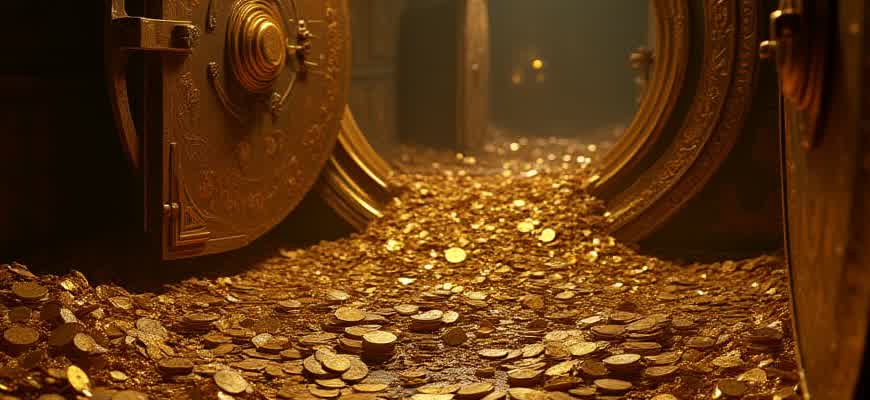
The integration of artificial intelligence (AI) into the cryptocurrency ecosystem is revolutionizing the way digital currencies are analyzed, traded, and secured. AI technologies are now being applied to streamline trading strategies, enhance security measures, and improve decision-making processes in crypto markets.
AI's role in the cryptocurrency domain can be segmented into various key functions:
- Predictive Analytics: AI models forecast market trends by analyzing vast amounts of historical data.
- Fraud Detection: Machine learning algorithms detect irregularities and prevent potential fraud in real-time transactions.
- Automation of Trading: Automated bots use AI to execute trades based on pre-programmed parameters, reducing human error.
- Smart Contracts: AI enhances smart contract performance by ensuring terms are met automatically without the need for intermediaries.
One of the most prominent applications of AI in crypto is the development of trading bots. These bots utilize machine learning algorithms to analyze data and execute trades at a speed and precision that human traders cannot match.
"AI-driven bots are capable of reacting to market changes in milliseconds, providing traders with a competitive advantage in volatile environments."
Key AI-driven tools include:
Tool | Description |
---|---|
AI Trading Bots | These bots use deep learning to make real-time trade decisions based on market sentiment and data trends. |
Fraud Prevention Algorithms | AI models that detect and prevent fraudulent activity by analyzing transaction patterns. |
Choosing the Optimal AI Model for Your Cryptocurrency Business
When integrating AI into a cryptocurrency business, selecting the right model can significantly impact both operational efficiency and market performance. Different AI models are designed for specific tasks such as market predictions, transaction fraud detection, or customer service automation. Therefore, understanding the nature of your business needs and matching them with the appropriate AI capabilities is crucial.
Before making a choice, businesses should assess various AI models based on the data they handle, the complexity of the processes they wish to optimize, and the scalability required for future growth. The effectiveness of AI largely depends on aligning it with the right business goals, ensuring that the model you choose can handle the specific demands of the crypto market.
Factors to Consider When Choosing an AI Model
- Data Availability: Ensure your business has access to the required data to train and optimize the AI model effectively.
- Model Accuracy: Evaluate the model’s ability to generate accurate predictions or decisions, which is vital in the highly volatile cryptocurrency market.
- Scalability: Consider whether the model can adapt as your business grows, especially if you plan to expand to new markets or handle increased transaction volumes.
- Customization Options: Look for models that offer flexibility, allowing customization to better meet the unique needs of your cryptocurrency platform.
Popular AI Models for Cryptocurrency Businesses
Model | Best For | Features |
---|---|---|
Neural Networks | Market Prediction, Risk Analysis | Deep learning, highly accurate for identifying patterns |
Decision Trees | Fraud Detection, Risk Management | Transparent decision-making, interpretable results |
Reinforcement Learning | Automated Trading Systems | Self-learning, adapts to market changes |
Remember, the right AI model can boost profitability by improving decision-making processes and automating complex tasks like market analysis and fraud detection in real time.
Understanding Machine Learning Algorithms in AI Solutions for Cryptocurrencies
Machine learning algorithms have become an integral part of cryptocurrency solutions, enabling sophisticated analysis and decision-making processes. These algorithms are used to predict market trends, optimize trading strategies, and even detect fraudulent activities. By analyzing large volumes of transaction data, machine learning models can uncover patterns that would otherwise be invisible to traditional methods.
The application of machine learning in cryptocurrency markets offers various benefits, such as real-time decision-making, adaptive trading strategies, and improved security. However, these systems are not without challenges, as the highly volatile nature of cryptocurrencies requires robust and dynamic models capable of adjusting to changing conditions.
Key Machine Learning Algorithms Used in Cryptocurrency Solutions
- Linear Regression: Often used for predicting price trends based on historical data.
- Random Forests: A machine learning technique that improves prediction accuracy by using multiple decision trees to identify market trends.
- Neural Networks: Applied to forecast future price movements and identify complex patterns in large datasets.
How Machine Learning Improves Cryptocurrency Security
One of the critical applications of machine learning in the cryptocurrency space is its role in enhancing security. By analyzing transaction data and network activity, machine learning algorithms can help detect irregular behavior and potential threats. Fraud detection systems powered by AI can identify suspicious transactions and flag them in real-time.
"Machine learning algorithms can significantly reduce the risk of fraud by analyzing vast amounts of data at speeds humans cannot match."
Challenges and Considerations
- Data Quality: The accuracy of predictions depends heavily on the quality of the input data. Inaccurate or incomplete data can lead to unreliable outcomes.
- Overfitting: Machine learning models may become too tailored to past data, making them ineffective in predicting future market behavior.
- Market Volatility: Cryptocurrencies are known for their high volatility, which makes it difficult for machine learning models to consistently generate accurate predictions.
Comparison of Popular Machine Learning Models
Model | Application | Strengths |
---|---|---|
Linear Regression | Price prediction | Simplicity and speed |
Random Forest | Trend analysis | High accuracy in complex datasets |
Neural Networks | Price forecasting and pattern recognition | Adaptability to dynamic market conditions |
Enhancing Customer Support in Cryptocurrency with Natural Language Processing
Natural Language Processing (NLP) has a significant role in transforming customer support systems, especially in the fast-paced world of cryptocurrencies. As the industry continues to grow, customer expectations for efficient, timely, and personalized support are at an all-time high. With the complexity of digital assets and blockchain technology, effective communication is essential to guide users through processes such as transactions, wallet management, and security concerns.
By implementing NLP tools, cryptocurrency platforms can offer more intuitive and responsive customer service. Through automated systems like chatbots, users can receive instant, context-aware support, reducing wait times and improving overall satisfaction. NLP allows the system to understand and process user queries accurately, even when phrased in diverse ways.
Key Benefits of NLP for Cryptocurrency Customer Support
- Improved Query Understanding: NLP allows systems to recognize complex and varied user requests related to cryptocurrency transactions, wallets, and market data.
- 24/7 Availability: Automated support systems can operate around the clock, ensuring that users receive assistance at any time, regardless of timezone differences.
- Personalized Responses: NLP systems can adapt to individual user preferences, offering tailored solutions based on previous interactions and transaction histories.
- Multilingual Support: With NLP's language capabilities, platforms can offer customer support in multiple languages, enhancing accessibility for global users.
Practical Applications
- Chatbots for Transaction Assistance: Automated assistants can help users track transaction status, troubleshoot issues, and even guide them through step-by-step processes in setting up wallets or trading assets.
- Security Support: NLP-powered tools can quickly identify potential security threats or phishing attempts by analyzing user queries and suggesting preventive actions.
- Knowledge Base Integration: NLP helps create smarter knowledge bases that offer precise, easy-to-understand answers to common cryptocurrency-related questions.
Example: Customer Query Handling
User Query | NLP Response |
---|---|
How do I recover my lost wallet password? | To recover your wallet password, follow the steps in the account recovery section. If you need further assistance, please verify your identity via email. |
Why is my transaction pending for so long? | Transaction delays can occur due to network congestion. Please wait for confirmation, or you may try adjusting the transaction fee for faster processing. |
Important: Implementing NLP in cryptocurrency support systems not only reduces operational costs but also provides a scalable solution for growing user bases, especially in high-demand environments like crypto exchanges.
Integrating AI with Cryptocurrency Business Models
Artificial intelligence (AI) is rapidly transforming the cryptocurrency industry, enhancing its ability to analyze massive datasets, predict market trends, and automate complex processes. AI integration allows businesses to improve transaction efficiency, reduce fraud risks, and optimize customer experiences. The challenge lies in seamlessly embedding AI solutions into the existing infrastructure of cryptocurrency exchanges, wallets, and trading platforms without disrupting core operations.
By leveraging AI, cryptocurrency businesses can gain valuable insights from market data, adapt to changing consumer behaviors, and streamline workflows. The combination of AI and blockchain technology can enable real-time monitoring, identify unusual patterns, and enhance the security of decentralized systems. Below are several key aspects to consider when integrating AI into a cryptocurrency business.
Key Considerations for AI Integration in Cryptocurrency
- Data Security: AI models require access to sensitive financial and transaction data. Ensuring data privacy and implementing robust cybersecurity protocols are critical to maintaining trust.
- Automation of Trading Algorithms: AI-powered trading bots can optimize asset management by making real-time decisions based on market fluctuations, providing a competitive edge.
- Fraud Detection and Prevention: AI tools can analyze transaction patterns to identify anomalies and predict fraudulent activities, significantly improving system security.
- Regulatory Compliance: Incorporating AI for compliance checks can help businesses stay aligned with global financial regulations, ensuring adherence to KYC (Know Your Customer) and AML (Anti-Money Laundering) laws.
Implementation Steps for AI Integration
- Assess the business needs and identify areas where AI can create the most value, such as predictive analytics or fraud prevention.
- Collaborate with AI technology providers to develop or customize models suited to the cryptocurrency ecosystem.
- Integrate AI solutions with blockchain systems, ensuring that they work seamlessly with the decentralized nature of cryptocurrency networks.
- Monitor and refine AI-driven processes based on real-time performance, adjusting for market volatility and emerging threats.
"Integrating AI with cryptocurrency platforms not only enhances operational efficiency but also strengthens security measures, ensuring better user trust and compliance with global financial standards."
AI Applications in Cryptocurrency: Example Table
AI Application | Impact |
---|---|
Automated Trading Bots | Increase trading efficiency, improve market predictions, and reduce manual errors. |
AI for Fraud Prevention | Early detection of unusual patterns and prevention of fraudulent transactions. |
AI-driven Risk Management | Better decision-making in volatile markets and minimized financial risks. |
Essential Tools for AI Integration in Crypto Data Analysis
Artificial Intelligence plays a crucial role in enhancing the capabilities of cryptocurrency data analysis. By leveraging advanced machine learning and deep learning models, AI enables more accurate predictions, automated trading strategies, and enhanced risk management. These tools help analysts and traders to process vast amounts of data in real-time, uncover hidden patterns, and optimize their investment decisions.
In the world of cryptocurrencies, where market volatility is a common concern, AI provides valuable insights for identifying trends and price movements. Several key tools and techniques are used to implement AI successfully in crypto data analysis. These range from statistical methods to more sophisticated neural networks and natural language processing (NLP) algorithms, all of which are critical for providing actionable intelligence from large datasets.
Popular Tools for Implementing AI in Cryptocurrency Data Analysis
- TensorFlow: An open-source library developed by Google, TensorFlow is widely used for building machine learning models, including neural networks and deep learning algorithms. It is highly flexible and scalable, making it suitable for complex cryptocurrency data tasks.
- PyTorch: PyTorch is another open-source machine learning framework used for research and production. Its dynamic computation graph is particularly useful for handling the large volumes of data found in crypto markets.
- Scikit-learn: A robust library for machine learning in Python, Scikit-learn is widely used for data analysis, including clustering, regression, and classification models.
- Natural Language Processing (NLP) Tools: NLP tools such as NLTK and spaCy are important for sentiment analysis, helping to understand market sentiment from news, tweets, and other social media sources.
Key Techniques in AI for Cryptocurrency Analytics
- Predictive Analytics: AI algorithms can predict future market trends based on historical data. Machine learning models, such as decision trees and regression analysis, can forecast the price movement of cryptocurrencies with remarkable accuracy.
- Algorithmic Trading: AI tools can be used to develop automated trading bots that execute trades based on predefined strategies. These bots use AI algorithms to detect market conditions in real-time and adjust trading strategies accordingly.
- Fraud Detection: AI-driven models help detect suspicious transactions, identify patterns of fraud, and assess potential threats to cryptocurrency security.
Important Considerations When Using AI for Crypto Data Analysis
Tool | Use Case | Advantages |
---|---|---|
TensorFlow | Building neural networks for price prediction | High scalability, supports deep learning |
PyTorch | Real-time data processing and prediction | Flexible, easy to debug, strong community |
Scikit-learn | Data analysis and basic machine learning tasks | Simple API, fast execution for smaller models |
"AI is not just a tool for enhancing efficiency; it is a game changer in how we interpret and act on cryptocurrency market data. With the right tools, traders can gain unprecedented insights and make smarter decisions."
Overcoming Challenges in Deploying AI Models for Crypto Markets
Deploying AI models within the cryptocurrency space presents several challenges, including data complexity, model scalability, and real-time performance. The decentralized and volatile nature of cryptocurrency markets requires AI models to handle a vast amount of dynamic data, such as price fluctuations, trading volumes, and social media sentiment. Overcoming these hurdles is essential for ensuring that AI models provide accurate insights and actionable recommendations for traders and investors.
To address the complexities of deploying AI in the crypto world, it's important to focus on optimizing data pipelines, enhancing model robustness, and ensuring smooth integration with trading platforms. Additionally, security measures must be a priority to protect both the model and the data. Below are key strategies and tools to tackle these challenges effectively.
Key Strategies for Overcoming Deployment Challenges
- Data Preprocessing: Ensuring that data is clean, normalized, and consistent is crucial. This involves removing outliers, handling missing data, and scaling features to improve model accuracy and stability in real-time environments.
- Model Optimization: To improve performance, techniques such as pruning, quantization, and knowledge distillation are used to reduce model size and improve speed without compromising accuracy.
- Real-Time Data Integration: Deploying AI models that can handle live, streaming data is key for cryptocurrency trading. This involves setting up real-time data pipelines using tools like Apache Kafka or RabbitMQ.
- Security Measures: Ensuring that models are secure from adversarial attacks and that the trading environment is safe from data breaches or model manipulation is critical in crypto markets.
Steps to Ensure Smooth AI Model Deployment in Cryptocurrency
- Testing in Simulated Environments: Before deploying in a live setting, AI models should be tested extensively in simulated trading environments to evaluate performance under different market conditions.
- Continuous Monitoring: After deployment, continuous monitoring is essential to track the model's performance and detect any degradation over time. Tools like Grafana or Prometheus can be used for real-time performance monitoring.
- Model Retraining: AI models must be regularly updated with new data to ensure they remain accurate in changing market conditions. Scheduled retraining ensures that models adapt to new trends and patterns in crypto trading.
Considerations When Implementing AI in Crypto Trading
Challenge | Solution | Tools |
---|---|---|
Data Quality | Clean and preprocess data effectively | Pandas, NumPy, Dask |
Model Latency | Optimize models for low-latency predictions | TensorFlow Lite, ONNX |
Security | Implement robust security measures | Secure model APIs, encryption techniques |
"Deploying AI in cryptocurrency trading requires not only technical expertise but also a deep understanding of market dynamics. A model that performs well in a lab may struggle in the unpredictable world of crypto if not carefully optimized and maintained."
Improving Cryptocurrency Trading Decisions with AI-Driven Predictive Analytics
In the fast-paced world of cryptocurrency, accurate decision-making is essential for gaining an edge over the competition. With the volatile nature of digital currencies, traditional methods of analyzing market trends may not be sufficient to predict future movements. This is where AI-powered predictive analytics comes into play, offering new tools and techniques to forecast market behavior more precisely.
AI algorithms can process vast amounts of data, identify complex patterns, and predict potential price movements in the crypto market. This ability significantly enhances decision-making processes for traders and investors, helping them act on insights that would be otherwise difficult to capture. By leveraging machine learning and historical data, predictive analytics improves the overall trading strategy.
Key Advantages of AI in Cryptocurrency Trading
- Enhanced Accuracy: AI models analyze multiple variables to predict market trends with greater precision than traditional methods.
- Faster Responses: AI systems can process data and react to changes instantly, allowing traders to capitalize on market shifts quickly.
- Emotionless Trading: AI removes human emotion from trading decisions, preventing impulsive actions and improving overall strategy consistency.
How Predictive Analytics Work in Cryptocurrency Markets
- Data Collection: AI gathers data from various sources such as historical prices, trading volumes, and social media sentiment.
- Pattern Recognition: Machine learning algorithms detect correlations and trends that humans might overlook.
- Forecasting: Based on identified patterns, predictive models forecast future price movements, assisting traders in making informed decisions.
"AI's ability to process and analyze large datasets in real-time gives cryptocurrency traders a competitive advantage, allowing for smarter investment decisions."
Example of Predictive Analytics in Action
Crypto Asset | Predicted Price Movement | Confidence Level |
---|---|---|
Bitcoin | Increase | 85% |
Ethereum | Decrease | 78% |
Litecoin | Stable | 90% |