How Are Ai Voices Made
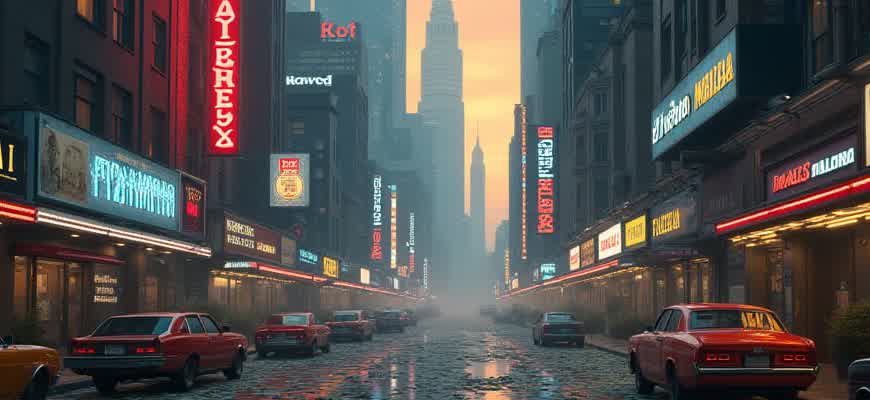
AI-generated voices have revolutionized the way we interact with digital systems. These voices are produced using complex algorithms and advanced neural networks, enabling machines to generate lifelike speech patterns that sound remarkably human-like. Below is an overview of the key steps involved in creating these voices.
- Data Collection: A vast database of human speech recordings is essential for training AI models. These recordings are carefully selected to capture various accents, tones, and speaking styles.
- Preprocessing: The raw audio data is cleaned and transformed into a format that the AI model can work with. This often involves isolating individual sounds, removing background noise, and normalizing volume levels.
- Model Training: Advanced machine learning techniques, such as deep learning and neural networks, are used to train the AI on how to produce natural-sounding speech. The model learns to map input text to the appropriate sounds and intonation.
Once the AI has been trained, it can generate voice outputs for any given input text by using a process called text-to-speech (TTS). Here’s a breakdown of the process:
- Text Analysis: The input text is parsed to understand its structure, including punctuation and grammar, which helps the AI determine the correct tone and rhythm.
- Phonetic Conversion: The text is then converted into phonetic representations–syllables and words that correspond to sounds.
- Speech Synthesis: Finally, the model synthesizes the speech by combining the learned phonetic patterns with prosodic features like pitch, speed, and emphasis.
"The accuracy of AI-generated voices heavily depends on the quality of training data and the sophistication of the underlying neural network architecture."
Step | Description |
---|---|
Data Collection | Gathering diverse and high-quality voice samples for training. |
Preprocessing | Cleaning and formatting audio data for machine learning. |
Model Training | Utilizing deep learning to train the AI on speech generation. |
How Cryptocurrencies and Blockchain are Impacting AI Voice Technology
In recent years, the integration of artificial intelligence (AI) and blockchain technology has transformed many industries, including the development of AI voices. The intersection of these two technologies opens up new possibilities for secure, decentralized voice generation systems. In the context of cryptocurrencies, AI-generated voices can enhance user interaction in decentralized platforms, where anonymity and security are prioritized.
The process of creating AI voices involves complex algorithms and machine learning models that require vast datasets. Cryptocurrencies, particularly their underlying blockchain technologies, have facilitated the creation of more transparent, trustworthy, and secure platforms for training these AI systems. This is particularly useful in environments where data privacy is a significant concern, such as in financial transactions or private blockchain-based communications.
Key Components of AI Voice Creation in Cryptocurrency Platforms
Creating AI voices is a multi-step process that blends advanced algorithms, neural networks, and natural language processing (NLP). Below are the fundamental elements involved:
- Data Collection: Massive datasets of human speech are used to train AI models. These datasets include a wide range of vocal tones, accents, and emotions.
- Model Training: Using deep learning, AI models are trained to recognize patterns and generate human-like speech. The more data available, the better the model's accuracy.
- Blockchain Integration: Blockchain networks offer a decentralized infrastructure for securely storing voice data and ensuring data privacy, which is critical in cryptocurrency environments.
Steps in AI Voice Generation Process
- Preprocessing: Raw audio data is cleaned and normalized before it’s used in training the AI models.
- Feature Extraction: Key characteristics of the voice, such as pitch, tone, and cadence, are extracted to teach the AI system how to replicate human speech.
- Model Deployment: Once trained, the AI model can generate speech in real-time, either for virtual assistants or automated customer service in cryptocurrency exchanges.
Benefits of Blockchain in AI Voice Systems
The integration of blockchain in AI voice technology has notable advantages, including:
Benefit | Description |
---|---|
Data Security | Blockchain ensures that the data used to train AI models is securely stored, preventing unauthorized access and tampering. |
Decentralization | By leveraging blockchain, AI voices can be generated and controlled in a decentralized manner, reducing the risk of centralized data breaches. |
Transparency | Transactions and voice data generation processes are transparent and auditable on a blockchain, fostering trust among users. |
"AI voice technology combined with blockchain is creating new, more secure, and decentralized ways of interacting with digital platforms, especially within the cryptocurrency world."
Understanding the Basics of AI Voice Technology
AI voice technology has evolved significantly, enabling systems to simulate human speech with a high degree of accuracy. It relies on sophisticated algorithms and neural networks to process and replicate various vocal characteristics. The process involves training AI models on vast datasets that consist of human speech samples, which are then used to generate synthetic voices. These systems are able to adapt to different languages, tones, and even emotional expressions, creating highly personalized and contextually relevant responses.
In the context of cryptocurrency, AI voices can be particularly useful in automating communication within platforms, enhancing user experiences, and improving accessibility. From customer support to educational content, AI-generated voices allow platforms to engage users in a more interactive and dynamic way. By integrating these technologies, cryptocurrency services can bridge the gap between complex financial systems and their users, providing information quickly and efficiently.
How AI Voices are Generated
The process of generating AI voices typically involves the following stages:
- Data Collection: Large amounts of voice data are gathered, including different accents, languages, and tones.
- Model Training: Neural networks are trained using the collected data to recognize patterns and generate natural-sounding voices.
- Text-to-Speech (TTS) Synthesis: Once trained, the AI can convert written text into spoken language in real-time.
Applications in the Cryptocurrency Space
AI-generated voices have several practical applications in the crypto industry:
- Automated Customer Support: AI voices can provide 24/7 assistance to users, answering questions and resolving issues without human intervention.
- Educational Content Delivery: Crypto platforms can use AI voices to explain complex financial concepts in an easy-to-understand manner.
- Real-Time Notifications: Users can receive instant updates about their cryptocurrency assets or trading activities in a conversational style.
Key Differences in AI Voice Systems
Type | Features | Use Case |
---|---|---|
Text-to-Speech (TTS) | Converts text into human-like speech | Customer support, news updates |
Speech Recognition | Converts spoken words into text | Voice commands, crypto transaction verification |
Note: As AI voices become more advanced, the boundary between synthetic and human speech continues to blur, opening up new possibilities for interaction in the digital space.
Choosing the Right Voice Data for Training AI Models in Cryptocurrency Industry
When developing AI models for voice synthesis, particularly in niche sectors like cryptocurrency, selecting the appropriate voice data becomes essential. A model trained on relevant, high-quality data ensures that it captures the nuances of industry-specific terminology and user intent. This is especially important for cryptocurrency, where the language evolves rapidly, and accurate communication of financial information is crucial.
The key challenge is to gather diverse and representative data that mirrors the specific needs of the cryptocurrency sector. This includes a variety of tones, speech patterns, and the inclusion of specialized terms that are common within this space. Without careful data selection, the AI model risks misinterpreting or misrepresenting the dynamic language used in cryptocurrency discussions.
Factors to Consider in Selecting Voice Data
- Domain-Specific Vocabulary: Ensure that the dataset includes crypto-related terms, such as "blockchain", "tokens", "decentralized", and "smart contracts". This helps the AI model accurately recognize and generate relevant speech.
- Speech Diversity: A varied dataset that includes different accents, age groups, and vocal tones helps in creating a model that is adaptable and realistic in real-world applications.
- Data Quality: High-quality recordings are essential. Background noise, poor clarity, or technical issues can negatively impact model performance.
Selecting the right voice data ensures that the AI model can engage with users effectively, whether in customer service, trading platforms, or education within the cryptocurrency space.
Recommended Data Collection Methods
- Professional Voice Talent: Work with professional voice actors familiar with cryptocurrency terminology to create a baseline dataset that reflects the industry's tone and complexity.
- Publicly Available Datasets: Use open-source datasets that include a variety of voices, but ensure they are carefully curated to include specific crypto-related phrases.
- User-Generated Content: Gather voice data from cryptocurrency forums, podcasts, and videos to include real-world examples of how individuals discuss the industry.
Example of Voice Data Selection Criteria
Criteria | Importance |
---|---|
Crypto Vocabulary | High |
Speech Clarity | High |
Diversity of Voices | Medium |
Accents and Dialects | Medium |
The Role of Deep Learning in Creating Natural-Sounding Voices
Deep learning has made significant advancements in recent years, particularly in the field of artificial intelligence voice generation. The technology has evolved from basic speech synthesis to highly realistic, human-like voices, with applications spanning from virtual assistants to voiceovers for cryptocurrency-related media. By leveraging large neural networks, deep learning models can now capture nuances such as tone, emotion, and cadence, which are essential for producing convincing voice outputs.
In the context of cryptocurrency, where communication is key to fostering trust and engagement, the ability to generate natural-sounding voices plays a pivotal role. Investors and users of crypto platforms are more likely to engage with content that feels authentic. The development of realistic voice models allows for more personalized interactions, whether in customer support systems or instructional videos explaining complex blockchain concepts.
Key Elements of Deep Learning for Voice Generation
- Neural Networks: These are the backbone of AI-driven voice synthesis. Networks are trained using vast datasets to learn patterns in speech.
- Speech Synthesis Models: Text-to-speech models are designed to convert text into natural speech by understanding phonetics and prosody.
- Recurrent Neural Networks (RNNs): These networks are particularly effective in understanding the sequential nature of language, crucial for coherent and fluid speech.
How Deep Learning Contributes to Voice Naturalness
- Training on Large Datasets: Deep learning models are trained on enormous amounts of voice data, which allows them to understand various accents, speech patterns, and even emotional tones.
- Context-Aware Synthesis: AI systems can now take into account the context of the conversation, making responses sound more natural and appropriate for the situation.
- Continuous Learning: These systems improve over time, learning from feedback to become even more accurate in replicating human speech.
"In cryptocurrency, trust is critical. Natural-sounding AI voices can bridge the gap between cold, mechanical systems and users seeking reliable, human-like interactions."
Comparison of Speech Models
Model | Technology | Key Feature |
---|---|---|
WaveNet | Deep neural networks | Produces highly realistic human speech with lifelike nuances. |
Tacotron 2 | Sequence-to-sequence model | Generates human-like speech from text with prosody and emotion. |
FastSpeech | Parallel text-to-speech system | Improves speech synthesis speed while maintaining natural quality. |
Steps to Train AI Models for Speech Synthesis in Cryptocurrency
Training AI models for speech synthesis involves a sequence of well-defined steps. In the context of cryptocurrency, these models are particularly useful for developing applications that generate natural-sounding voices for virtual assistants, announcements, or educational content related to blockchain technology. The process begins with data collection, followed by pre-processing, model training, and fine-tuning to ensure the output mimics human speech with high accuracy.
For cryptocurrency platforms, implementing speech synthesis can significantly enhance user engagement. This can involve converting news about crypto market movements, providing audio versions of trading guides, or even generating automated reports on token performance. The AI models used for this are typically trained on extensive datasets, with particular attention paid to the nuances of speech that can reflect the tone of market trends or financial updates.
Key Steps in AI Speech Synthesis Training
- Data Collection: Gather diverse voice samples, including different accents and tones, to train the model effectively.
- Pre-processing: Clean and segment audio data to align speech and text pairs.
- Feature Extraction: Analyze and extract relevant features from the audio, such as pitch, speed, and frequency.
- Model Training: Utilize deep learning models like WaveNet or Tacotron to generate synthesized speech based on the extracted features.
- Post-processing: Enhance the audio quality and refine output to sound more natural and less robotic.
For cryptocurrency projects, it’s essential that the speech synthesis model understands jargon and terminologies unique to the market, such as "blockchain," "mining," "tokens," and "decentralized." Proper training ensures that these terms are pronounced correctly.
Training Frameworks and Tools
Framework | Features | Usage in Crypto Applications |
---|---|---|
TensorFlow | Open-source, scalable machine learning platform for building deep learning models | Widely used for training neural networks for speech synthesis in crypto platforms |
PyTorch | Dynamic computation graphs, popular in academic research and development | Used for rapid experimentation and fine-tuning speech models with specialized crypto terminology |
FastSpeech | Optimized for faster and more efficient speech synthesis | Efficient for real-time cryptocurrency notifications and voice alerts |
Fine-Tuning AI Voices for Varied Emotional Tones in Crypto Communication
In the world of cryptocurrency, clear and engaging communication is vital, especially as the industry continues to expand globally. As AI-generated voices become more common in delivering financial insights and updates, their ability to reflect the proper emotional tone can significantly enhance user experience and decision-making. Tailoring the voice's emotional range to fit specific market conditions–be it bullish excitement or cautious bearishness–can directly impact the perception of the message being conveyed.
To fine-tune AI voices for emotional resonance in the context of cryptocurrency, developers use advanced techniques that allow for adjusting pitch, speed, and tone. This customization ensures that investors or traders hear messages that align with the mood of the market, promoting clearer comprehension and appropriate action.
Techniques for Emotional Customization in AI Voices
- Pitch Adjustments: Altering the pitch helps convey different emotional states such as urgency or calmness. Higher pitches often evoke excitement, while lower pitches signal stability or caution.
- Speed Modulation: Changing the speaking rate can make the voice seem more relaxed or urgent, depending on the sentiment needed for the moment–whether it’s a rapid market update or a steady explanation of a long-term investment strategy.
- Voice Characterization: Using specific tonal qualities tailored to the crypto space, such as neutral or authoritative tones, ensures that messages are perceived as trustworthy, important, or even motivational.
Fine-tuning the emotional tone of AI voices allows for better engagement with cryptocurrency users, ensuring that the voice aligns with the intended message, whether optimistic or cautious.
Example of Emotional Adjustments for Crypto Communications
Market Condition | Emotional Tone | Voice Adjustment |
---|---|---|
Bullish Trend | Excited, Optimistic | Higher pitch, faster speed |
Bearish Trend | Calm, Cautious | Lower pitch, moderate speed |
Neutral Market | Informative, Neutral | Medium pitch, steady pace |
How Voice Data is Processed for AI Speech Synthesis
In the world of AI voice generation, raw speech samples undergo a rigorous process to be converted into usable data for creating realistic voice models. This process is crucial for applications in fields like cryptocurrency trading platforms, where natural-sounding voice assistants help users navigate complex market data. The main challenge is ensuring that the voice retains human-like characteristics while being clear and intelligible in different contexts, such as technical jargon or financial terminology.
AI speech models are trained using vast amounts of voice recordings that capture diverse phonetic and tonal variations. These recordings are then processed into features that allow the AI to replicate not only speech patterns but also the nuances that define human communication, such as tone, pitch, and rhythm. The conversion of this data into understandable outputs is an essential part of creating realistic voice assistants that can interact with cryptocurrency market enthusiasts.
Steps in Processing Speech Data for AI Voice Models
- Data Collection: High-quality, diverse speech samples are gathered to create a wide range of phonetic and emotional contexts.
- Preprocessing: The raw audio data is cleaned, removing background noise and enhancing clarity.
- Feature Extraction: Key speech characteristics such as pitch, tone, and cadence are extracted and analyzed to build a model.
- Model Training: The extracted features are used to train machine learning models to understand and replicate speech patterns.
- Post-Processing: The generated voice is fine-tuned for naturalness, adjusting volume, pace, and pauses.
"The key to a successful AI voice lies in how accurately it mirrors human speech patterns, down to the subtlest nuances, enabling seamless interactions in complex environments like cryptocurrency trading platforms."
Key Factors in Voice Model Development
Factor | Description |
---|---|
Speech Diversity | Wide range of voices and accents are needed to ensure flexibility in real-world applications. |
Realism | The ability to recreate natural speech patterns, including pauses and emotional expressions. |
Context Sensitivity | The AI must adapt its speech style depending on the context, such as trading market updates versus casual conversation. |
Implementing AI Voices in Various Applications: From Apps to Virtual Assistants
AI-powered voices have transformed the way we interact with technology, enhancing user experiences in various sectors. In the world of cryptocurrency, these voices are integrated into platforms to provide interactive customer support, simplify trading processes, and enable seamless navigation through digital assets. With the rapid growth of cryptocurrency platforms, the use of AI voices ensures that users have an accessible and intuitive experience, making the technology more user-friendly, especially for newcomers.
As cryptocurrency applications evolve, AI voices are becoming an essential tool for improving customer engagement. AI systems can interpret market trends, respond to user queries, and even provide real-time updates on coin prices. By integrating these voices into applications, users can now navigate complex financial systems with ease, empowering them to make informed decisions quickly. This shift is also helping bridge the gap between traditional finance and the emerging world of digital currencies.
Key Benefits of AI Voices in Crypto Applications
- Improved User Accessibility: AI voices provide an alternative to text-based interactions, allowing users to interact more intuitively with cryptocurrency platforms.
- Real-Time Assistance: With AI voices, users can receive instant updates on market prices, wallet balances, and investment insights.
- Personalized Experience: AI voices can tailor recommendations and responses based on user behavior and preferences, offering a more engaging experience.
Challenges of Implementing AI Voices in Crypto Platforms
- Accuracy of Information: Ensuring the AI voice provides correct and up-to-date financial data is crucial, as users rely on it for making significant investment decisions.
- Security Concerns: Given the sensitive nature of cryptocurrency transactions, integrating AI voices must prioritize user security to prevent fraud or data breaches.
- Language Barriers: With a global audience, it's important for AI systems to support multiple languages and accents to serve users from diverse regions.
"AI voices in cryptocurrency applications have the potential to enhance user trust and simplify the complexities of digital asset management, but it's vital to address concerns around data security and system reliability."
Example Use Case: AI Voice for Cryptocurrency Trading Platforms
Feature | Impact |
---|---|
Voice-Activated Transactions | Allows users to initiate transactions through simple voice commands, making trading faster and more efficient. |
Market Trend Analysis | AI voices can read out complex market analysis, helping traders stay informed without needing to constantly monitor charts. |
Instant Notifications | Real-time alerts on significant market changes can be communicated directly via AI voices, providing immediate updates. |