Ai Based Sentiment Analysis Project
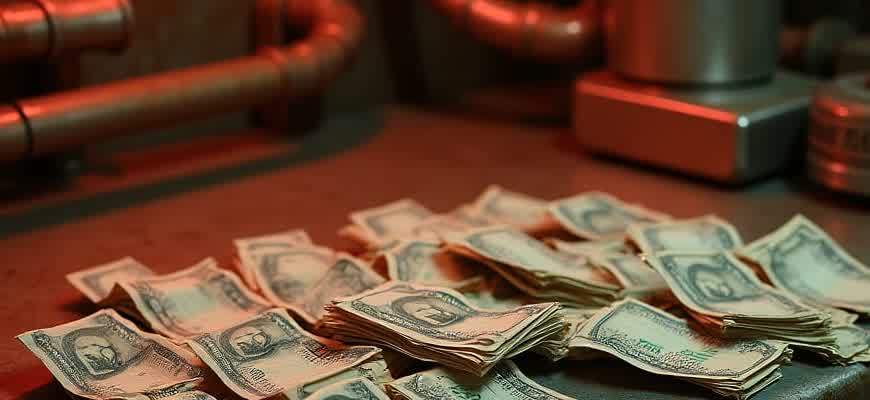
In the fast-paced world of cryptocurrencies, sentiment analysis has emerged as a crucial tool for understanding market trends and predicting price movements. By leveraging AI algorithms, we can analyze vast amounts of social media posts, news articles, and forum discussions to gauge investor sentiment. This provides a clearer picture of how market participants are feeling and reacting to news, which can help in making informed investment decisions.
The core of sentiment analysis involves the extraction of emotional tone from text data. AI models, particularly those based on natural language processing (NLP), can classify content as positive, negative, or neutral. This classification is then used to predict short-term market behavior, providing an edge in the highly volatile cryptocurrency environment.
"AI sentiment analysis offers a powerful way to understand investor psychology in real-time, allowing traders to make data-driven decisions."
- Real-time market monitoring
- Analysis of social media trends
- News sentiment tracking
- Improved predictive models for price forecasting
Key Benefits:
- Enhanced market prediction accuracy
- Identification of potential investment opportunities
- Risk mitigation through sentiment tracking
Method | Accuracy | Timeframe |
---|---|---|
Social Media Monitoring | High | Short-Term |
News Article Sentiment | Medium | Mid-Term |
Forum Discussion Sentiment | Moderate | Long-Term |
AI-Powered Sentiment Analysis for Cryptocurrency: Gaining Business Insights
Cryptocurrency markets are notoriously volatile, driven by rapid shifts in investor sentiment. Analyzing social media trends, news articles, and community discussions can provide valuable insights into market movements. AI-based sentiment analysis tools have emerged as essential resources for businesses looking to make informed decisions in this space. By leveraging natural language processing (NLP) and machine learning models, these tools can process massive volumes of unstructured data to gauge public sentiment towards specific digital assets.
The AI-driven sentiment analysis approach can be particularly effective in the cryptocurrency world, where real-time reactions and emotions of traders and investors often dictate market trends. This allows businesses to predict price fluctuations and market sentiment, giving them a competitive edge. It also opens up new opportunities for marketing strategies and product positioning within the crypto ecosystem.
How AI Sentiment Analysis Works in the Cryptocurrency Market
- Data Collection: AI systems scrape vast amounts of data from forums, blogs, social media platforms like Twitter, Reddit, and news websites.
- Data Preprocessing: The collected data is cleaned and transformed into a suitable format for analysis, removing noise and irrelevant information.
- Sentiment Classification: Using advanced NLP techniques, AI categorizes sentiment as positive, neutral, or negative based on the content of the text.
- Market Trend Prediction: Sentiment analysis helps in predicting price trends by correlating sentiment scores with historical market data.
AI-powered sentiment analysis gives companies an edge by enabling data-driven decisions and uncovering hidden patterns in the market.
Real-World Applications in Cryptocurrency
- Investor Sentiment Tracking: AI can identify shifts in investor mood, providing early indicators of price swings.
- Risk Management: By understanding the prevailing sentiment, businesses can better anticipate potential risks and market crashes.
- Brand Positioning: Crypto businesses can use sentiment analysis to gauge public perception of their brand and tailor marketing efforts accordingly.
Impact on Cryptocurrency Trading
Sentiment Category | Market Reaction |
---|---|
Positive Sentiment | Increase in buying activity, potential price rise |
Negative Sentiment | Increase in selling activity, potential price drop |
Neutral Sentiment | Market stability, low volatility |
How AI Sentiment Analysis Improves Cryptocurrency Feedback Interpretation
Sentiment analysis plays a crucial role in understanding customer perspectives in the fast-evolving world of cryptocurrency. By applying AI-driven sentiment analysis techniques, businesses can decode user feedback and market sentiment more efficiently than traditional methods. In the volatile crypto market, where emotions often drive decision-making, this technology allows companies to swiftly adapt and refine their strategies. AI models can analyze vast amounts of feedback from social media, forums, and customer reviews, offering deep insights into public perception of specific cryptocurrencies or platforms.
As the cryptocurrency market experiences rapid changes, AI-powered sentiment analysis helps crypto-related businesses identify underlying patterns in consumer opinions. This approach not only enhances the understanding of user sentiment but also allows for better-targeted communications, risk management, and product development. By automating feedback analysis, businesses can quickly address emerging issues, detect fraud, and even predict market shifts based on customer attitudes towards new trends or regulatory changes.
Benefits of AI Sentiment Analysis in Crypto
- Real-time insights: AI tools can analyze large datasets instantly, providing businesses with up-to-date sentiment scores.
- Improved decision-making: Understanding public perception allows businesses to adjust their strategies, such as marketing campaigns or product offerings, based on current sentiment.
- Risk mitigation: AI models can detect negative sentiment or signs of fraud early, helping to manage risks before they escalate.
Challenges Addressed by AI Sentiment Analysis
- Data overload: Cryptocurrencies generate massive volumes of data daily. AI can process and filter this information efficiently, saving time and resources.
- Emotional bias: Human analysis often overlooks subtle emotional nuances. AI systems can detect sarcasm, anger, and excitement in feedback, ensuring more accurate sentiment interpretation.
- Market volatility: Cryptocurrencies are prone to rapid changes. AI can track shifts in sentiment and offer predictive insights on market movements, allowing businesses to react proactively.
Practical Example of AI Sentiment Analysis in Crypto
Sentiment Type | Customer Feedback | AI-Generated Insight |
---|---|---|
Positive | "I love how easy it is to trade Bitcoin now!" | AI detects optimism about the ease of trading, suggesting potential for increased user acquisition efforts. |
Negative | "The recent security breach has made me cautious about using this platform." | AI identifies concern over security, recommending immediate communication and transparency to address user concerns. |
AI sentiment analysis transforms how crypto platforms interpret user feedback, enabling them to make informed decisions that enhance customer satisfaction and market positioning.
Leveraging AI for Cryptocurrency Sentiment Analysis on Social Media
The volatile nature of cryptocurrencies makes them highly sensitive to public sentiment. Real-time analysis of social media platforms like Twitter, Reddit, and Telegram can provide invaluable insights into the mood of investors, traders, and the general public. By applying artificial intelligence, it is possible to track and analyze the sentiment surrounding various cryptocurrencies, helping to predict market movements and identify emerging trends. With AI-driven sentiment analysis tools, it becomes easier to differentiate between positive, negative, and neutral sentiments in social media posts related to specific digital assets.
AI can be integrated into social media sentiment analysis through natural language processing (NLP) and machine learning models. These technologies can process massive volumes of unstructured data, detect underlying emotions, and provide actionable insights. By continuously monitoring platforms, AI systems can also identify early signs of market shifts, enabling traders to adjust their strategies in real time. This type of monitoring is crucial in the highly dynamic and fast-paced cryptocurrency market.
Key Steps in Implementing AI for Social Media Sentiment Monitoring
- Data Collection: Gather social media posts from platforms like Twitter, Reddit, and specialized crypto forums.
- Preprocessing: Clean and prepare the data by removing irrelevant content, links, and stop words.
- Sentiment Classification: Use machine learning models to classify posts as positive, negative, or neutral.
- Real-Time Monitoring: Implement real-time data collection and analysis to track market sentiment changes instantly.
- Visualization: Display sentiment trends on dashboards, allowing users to interpret data effectively.
Important Note: Real-time sentiment analysis can offer immediate insights but is not a substitute for in-depth market analysis. It should be used as a complementary tool for making trading decisions.
Comparison of AI Models for Cryptocurrency Sentiment Analysis
Model | Strengths | Weaknesses |
---|---|---|
Sentiment Polarity Analysis | Easy to implement, effective for detecting basic sentiment trends | Limited understanding of sarcasm and mixed emotions |
Deep Learning Models | Highly accurate, capable of understanding complex sentiment | Require large datasets and significant computational power |
Lexicon-based Sentiment Analysis | Good for small datasets, interpretable results | Limited flexibility, cannot detect nuances or evolving language |
By selecting the appropriate AI model for sentiment analysis, cryptocurrency traders and investors can harness social media data to gain an edge in predicting market sentiment and trends. As AI technology continues to advance, its role in crypto market analysis will only grow more significant.
Optimizing Product Development with AI-Driven Sentiment Data in Cryptocurrency
In the rapidly evolving cryptocurrency market, product development must be agile and responsive to shifting user sentiments. AI-powered sentiment analysis tools are proving invaluable for understanding customer opinions and market trends. These insights allow developers and product teams to tailor their offerings to meet user expectations and address potential issues before they escalate. By analyzing user feedback across social media platforms, forums, and transaction histories, companies can gauge the overall mood and sentiment surrounding their products, enabling them to refine their strategies in real time.
AI sentiment analysis also helps to identify patterns that might otherwise go unnoticed in the high-velocity cryptocurrency sector. By processing large datasets, AI can detect emerging trends and provide actionable insights, allowing companies to make informed decisions quickly. This approach not only enhances the user experience but also drives innovation, helping firms stay ahead of competitors. Integrating sentiment data into the product development cycle can lead to more tailored features, increased user satisfaction, and a stronger market position.
Key Benefits of Using Sentiment Data for Product Development
- Market Adaptation: AI sentiment analysis allows for real-time feedback, enabling developers to quickly pivot product features based on user preferences.
- Risk Mitigation: By detecting negative sentiment early, companies can address issues proactively, minimizing potential damage to their reputation.
- Feature Optimization: Insights from sentiment data help in fine-tuning features, ensuring they meet user needs and align with market demands.
By leveraging AI-driven sentiment analysis, cryptocurrency companies can enhance their product development cycles, ensuring products are not only innovative but also deeply aligned with the needs and desires of their user base.
How Sentiment Data Can Influence Cryptocurrency Product Features
- Identifying Popular Features: AI can highlight which features users are discussing positively, suggesting where to focus development efforts.
- Improving Customer Experience: Negative sentiment analysis helps identify pain points, enabling teams to resolve issues that hinder user satisfaction.
- Anticipating Market Shifts: Monitoring shifting sentiments can alert teams to emerging trends or potential downturns, helping to adjust the product roadmap accordingly.
Sentiment Type | Impact on Product Development |
---|---|
Positive | Highlights features and aspects that resonate well with users, guiding future feature enhancements. |
Negative | Indicates areas of dissatisfaction, leading to targeted improvements and troubleshooting. |
Neutral | Provides general feedback that can guide minor tweaks and updates. |
Leveraging AI-Driven Sentiment Analysis for Enhancing Cryptocurrency Customer Support
As the cryptocurrency market continues to expand, customer support for crypto-related services faces the challenge of dealing with vast amounts of user feedback. AI-powered sentiment analysis tools provide an effective way to address this issue by automatically assessing customer emotions and feedback on platforms. By integrating these tools into customer service systems, crypto platforms can respond faster and more efficiently to user inquiries, ensuring a more personalized and accurate support experience.
Sentiment analysis allows platforms to detect underlying feelings, whether positive, negative, or neutral, within customer messages. This enables customer service teams to prioritize urgent issues, identify areas of dissatisfaction, and provide tailored solutions, especially during market volatility or technical issues. Here’s how sentiment analysis can be integrated into crypto service platforms:
- Instant Feedback Analysis: AI systems can evaluate customer interactions in real-time, detecting sentiments like frustration or confusion, allowing for prompt responses.
- Improved Issue Prioritization: By understanding the emotional tone of customer queries, platforms can prioritize issues based on urgency or severity.
- Enhanced User Experience: A sentiment-aware support system can adjust its responses according to the user's emotional state, leading to a more empathetic and supportive interaction.
Key Benefits of AI Sentiment Analysis for Cryptocurrency Platforms:
Benefit | Impact |
---|---|
Faster Response Times | By identifying critical issues through sentiment analysis, customer service teams can respond more promptly. |
Data-Driven Insights | AI-driven sentiment tools can provide valuable analytics for improving platform features and user interactions. |
Proactive Support | Sentiment analysis helps identify users at risk of frustration, enabling preemptive actions to prevent dissatisfaction. |
"AI tools not only streamline customer support but also help create deeper, more meaningful relationships with users by responding to their emotional needs in real-time."
Training Your AI Model for Cryptocurrency Sentiment Analysis: Best Practices
Building an AI model that accurately detects sentiment in cryptocurrency-related content requires careful consideration of various factors. The volatile and ever-evolving nature of the crypto market makes sentiment analysis especially challenging. Whether the content is news articles, social media posts, or market analysis, it is crucial to understand how the context and language used by the crypto community can influence sentiment. By following a structured approach to training your AI model, you can enhance its ability to detect positive, negative, or neutral emotions within cryptocurrency discussions.
To ensure your model's success, selecting the right data sources and implementing proper preprocessing techniques are key. Using high-quality, relevant data will allow the model to learn from real-world crypto sentiments. Additionally, fine-tuning the model regularly as market trends shift will help maintain accuracy. Below are some best practices for training your AI model in the realm of cryptocurrency sentiment analysis.
Best Practices for Effective Cryptocurrency Sentiment Detection
- Data Selection: Focus on gathering data from diverse, high-quality sources such as social media platforms (e.g., Twitter, Reddit), crypto forums, and market news sites.
- Preprocessing: Clean and normalize text data by removing unnecessary symbols, correcting misspellings, and dealing with slang or abbreviations specific to the crypto community.
- Labeling Data: Ensure that your training data is correctly labeled with sentiment (positive, negative, neutral). This can be done manually or through semi-supervised methods.
- Feature Engineering: Use relevant linguistic features such as hashtags, mentions, and keywords that are common in crypto discussions. This can improve the accuracy of sentiment detection.
- Model Selection: Experiment with different machine learning algorithms such as LSTM (Long Short-Term Memory), BERT, or GPT-based models tailored for sentiment analysis tasks.
Evaluating Model Performance
- Cross-validation: Implement k-fold cross-validation to ensure that your model generalizes well and doesn’t overfit to specific datasets.
- Metrics: Use precision, recall, and F1-score to evaluate the model’s performance in detecting sentiment correctly.
- Continuous Monitoring: Regularly evaluate and fine-tune the model based on its real-world performance and feedback.
Regularly updating your model with fresh data and retraining it on new trends in cryptocurrency discussions is crucial. The crypto market evolves quickly, and so should your sentiment analysis model.
Model Performance Comparison
Model | Accuracy | Precision | Recall |
---|---|---|---|
LSTM | 85% | 88% | 83% |
BERT | 90% | 92% | 89% |
GPT-based | 87% | 89% | 85% |
How to Select the Best AI Sentiment Analysis Tool for Your Crypto Business
In the rapidly evolving world of cryptocurrency, sentiment analysis is becoming an essential tool for making informed business decisions. AI-powered sentiment analysis tools help crypto traders, investors, and companies assess market emotions, predict price movements, and understand public perception. Selecting the right tool requires understanding key features and capabilities that align with your business goals and data sources.
When choosing a sentiment analysis solution for the crypto market, it's vital to consider how the tool integrates with real-time data, its ability to analyze news, social media, and other cryptocurrency-related content, and its accuracy in predicting market shifts. Below, we’ll explore the factors you should prioritize in making this decision.
Key Factors to Consider
- Data Sources: The tool should be able to aggregate data from multiple relevant sources such as crypto news outlets, forums, social media platforms, and market reports.
- Real-time Analysis: Given the volatility of the cryptocurrency market, the analysis must be conducted in real-time to stay ahead of rapid changes.
- Accuracy of Sentiment Detection: Choose a tool that can differentiate between positive, neutral, and negative sentiments with a high degree of accuracy to prevent misinterpretation of data.
- Customizable Features: The tool should allow customization to focus on specific cryptocurrencies or particular market events relevant to your business.
Steps to Choose the Right Tool
- Evaluate Your Needs: Determine whether you need a basic sentiment analysis or a more advanced system capable of predictive analytics.
- Test Multiple Tools: Use trial versions to compare the tools' effectiveness in analyzing cryptocurrency market trends.
- Consider Integration Capabilities: Check if the tool can integrate with other platforms or tools you are already using for crypto trading or analysis.
“Sentiment analysis is a powerful tool for predicting crypto market shifts, but only if the data is accurate and up-to-date. Be sure to evaluate any tool thoroughly before making a commitment.”
Comparison of Top Sentiment Analysis Tools for Cryptocurrency
Tool Name | Data Source Coverage | Accuracy | Real-Time Analysis |
---|---|---|---|
CryptoSentiment Pro | News, Social Media, Forums | 90% | Yes |
SentimentX | News, Twitter, Reddit | 85% | No |
CryptoMood | News, Social Media, Market Reports | 92% | Yes |
Scaling Your Sentiment Analysis Project: Infrastructure and Costs
When developing a sentiment analysis project focused on cryptocurrency, one of the critical challenges is scaling the infrastructure to handle an increasing volume of data. Cryptocurrency markets are highly volatile, generating vast amounts of real-time information across social media, news platforms, and forums. This influx requires a robust system capable of processing and analyzing large datasets efficiently while maintaining speed and accuracy.
To scale such a project, it’s crucial to plan for both the computational resources and the associated costs. The infrastructure needs to handle multiple data sources, real-time analysis, and model training, all of which can put a strain on servers and storage solutions. As the project grows, ensuring the system can handle spikes in demand without affecting performance is essential for maintaining reliable sentiment analysis outputs.
Key Considerations for Scaling Your Sentiment Analysis Project
- Data Storage and Management: As the volume of cryptocurrency-related data increases, investing in scalable storage solutions like cloud services is essential. Distributed databases can handle large datasets while ensuring quick retrieval and redundancy.
- Compute Power: The need for powerful servers to process and analyze data in real-time is paramount. Cloud computing services (e.g., AWS, Google Cloud) offer scalable compute resources that can adjust as workload requirements grow.
- Model Optimization: Continuously improving the sentiment analysis model is essential for maintaining its accuracy as market trends evolve. This includes tuning the machine learning algorithms and retraining them with new data regularly.
"Scaling a sentiment analysis project for cryptocurrency requires a delicate balance between high-performance computing resources and cost efficiency."
Cost Breakdown for Scaling
Scaling the infrastructure for sentiment analysis comes with significant costs. These costs primarily arise from three key areas:
Category | Cost Factors |
---|---|
Data Storage | Cloud-based solutions, data redundancy, backup services |
Compute Power | On-demand cloud instances, data processing speed, server uptime |
Model Development | Machine learning tools, frequent retraining, algorithm improvements |
"Understanding the cost implications of scaling is crucial for making informed decisions that ensure long-term sustainability."