Knowledge Based Sentiment Analysis
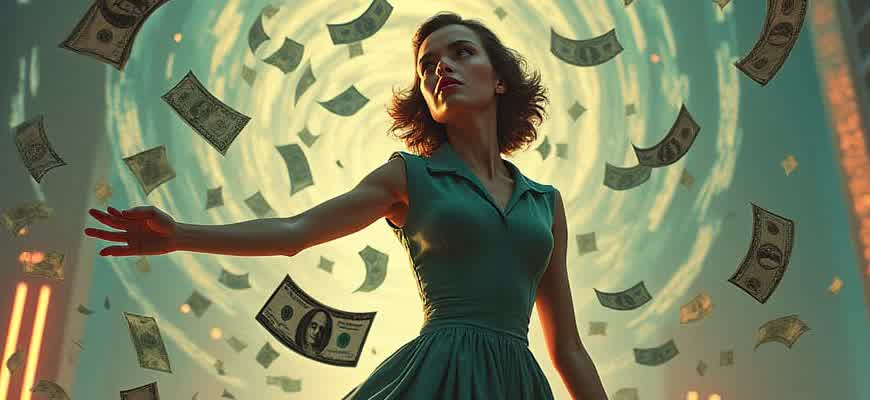
In the rapidly evolving cryptocurrency market, understanding public sentiment is crucial for predicting price movements and market trends. Traditional sentiment analysis techniques rely on raw data such as social media posts and news articles. However, a knowledge-based approach takes it further by integrating domain-specific expertise, helping analysts interpret the nuances behind market sentiment more accurately.
Key Elements of Knowledge-Based Sentiment Analysis:
- Contextual Understanding: By incorporating knowledge from financial and blockchain domains, the analysis becomes more relevant and precise.
- Data Sources: News feeds, social platforms, and expert opinions are processed using advanced Natural Language Processing (NLP) techniques.
- Behavioral Insights: Examining the reactions of market participants in response to news events provides deeper insights into sentiment shifts.
Knowledge-based sentiment analysis goes beyond surface-level emotions by adding context, improving the predictive power of market analysis.
To illustrate how this method is applied, here is a comparison of basic sentiment analysis versus knowledge-enhanced sentiment analysis:
Aspect | Basic Sentiment Analysis | Knowledge-Based Sentiment Analysis |
---|---|---|
Data Source | General Social Media, News Articles | Specialized Financial and Blockchain Data, Expert Opinions |
Accuracy | Moderate | High |
Contextual Relevance | Low | High |
Enhance Your Crypto Business with Sentiment Insights
In the volatile world of cryptocurrency, staying ahead of market trends is crucial for success. By leveraging knowledge-based sentiment analysis, businesses can make more informed decisions by tapping into the emotional and psychological responses of the market. This powerful tool helps in understanding public perception, identifying potential risks, and spotting emerging opportunities in real-time.
Knowledge-based sentiment analysis combines advanced data mining techniques with domain-specific knowledge to provide accurate and actionable insights. This approach not only focuses on the language of social media posts and news articles but also incorporates expert knowledge of the cryptocurrency landscape, allowing businesses to refine their strategies and enhance decision-making.
Key Benefits of Knowledge-Based Sentiment Analysis in Crypto
- Predictive Market Trends: By analyzing sentiment patterns, businesses can anticipate shifts in market behavior before they happen.
- Risk Mitigation: Sentiment analysis highlights potential market downturns or regulatory changes based on public sentiment, enabling timely risk management.
- Targeted Marketing Strategies: Understanding the emotions and opinions of specific crypto communities allows businesses to create tailored marketing campaigns.
How to Implement Sentiment Analysis in Crypto Trading
- Data Collection: Gather data from multiple sources, such as Twitter, Reddit, and news outlets, to get a holistic view of the market sentiment.
- Natural Language Processing (NLP): Use NLP algorithms to process and classify the emotions embedded in the data.
- Domain Knowledge Integration: Incorporate cryptocurrency-specific knowledge into the analysis for more accurate results.
- Continuous Monitoring: Regularly track sentiment shifts to stay ahead of market changes and adjust business strategies accordingly.
“Incorporating sentiment analysis into crypto trading can make the difference between being reactive and proactive in a fast-paced market.”
Example of Sentiment Trends in the Crypto Market
Sentiment Indicator | Impact on Market |
---|---|
Positive Sentiment Surge | Price increases and higher trading volume due to optimism about new regulations or advancements. |
Negative Sentiment Shift | Potential price dips and reduced market confidence as concerns over security or government intervention grow. |
Enhancing Customer Insights Through Knowledge-Based Sentiment Analysis in Cryptocurrency
In the cryptocurrency market, understanding consumer emotions and perceptions is crucial for predicting trends, driving adoption, and shaping business strategies. Knowledge-based sentiment analysis leverages industry-specific knowledge and predefined lexicons to better interpret user feedback and sentiments surrounding digital assets. By focusing on the context of blockchain technology, digital currencies, and market dynamics, this approach provides more accurate insights compared to traditional sentiment analysis techniques. The technology can track and analyze public discourse around major cryptocurrencies like Bitcoin, Ethereum, or emerging altcoins, offering valuable insights into market sentiments.
This method of sentiment analysis employs specialized algorithms and a deep understanding of the cryptocurrency ecosystem, including jargon, regulatory discussions, and market trends. The results can help identify shifts in investor confidence, market volatility, and potential opportunities or risks. By analyzing social media conversations, news articles, and online forums, knowledge-based sentiment analysis refines its results to deliver actionable insights that can inform investment decisions, customer engagement strategies, and even the development of new blockchain projects.
How Knowledge-Based Sentiment Analysis Provides Value
- Improved Accuracy: By incorporating specialized knowledge, sentiment analysis can distinguish between positive, neutral, or negative sentiments more effectively, even in complex cryptocurrency-related discussions.
- Predictive Analytics: Sentiment trends can offer early signals of market movements or investor sentiment, helping businesses and traders anticipate potential price fluctuations.
- Targeted Marketing: By understanding consumer attitudes, brands can tailor their marketing campaigns to address concerns, highlight strengths, and engage with their audience on a deeper level.
Key Benefits in Cryptocurrency Market
Benefit | Description |
---|---|
Informed Decision Making | Organizations can make better strategic choices, whether it’s investing in certain assets or adjusting their product offerings. |
Enhanced Customer Experience | By identifying pain points and customer needs, businesses can improve their services, resulting in increased customer satisfaction. |
Competitive Advantage | Firms gain a competitive edge by staying ahead of market trends and understanding what drives user behavior in the cryptocurrency space. |
"Incorporating knowledge-based sentiment analysis can significantly enhance how businesses interpret and act upon public perception, especially in volatile markets like cryptocurrency."
Integrating Knowledge-Based Sentiment Analysis into Your Existing CRM System
In the rapidly evolving cryptocurrency market, staying ahead of customer sentiment can provide invaluable insights for decision-making. Integrating sentiment analysis directly into your CRM system allows for real-time tracking of customer opinions, reviews, and discussions. This can significantly enhance customer engagement, personalize marketing efforts, and refine product offerings based on the latest market feedback.
By leveraging knowledge-based sentiment analysis, you can analyze social media posts, customer support interactions, and user-generated content to gauge public opinion about specific cryptocurrencies. This approach doesn’t rely solely on keyword matching but uses contextual understanding and predefined knowledge bases to assess sentiment more accurately. Integrating such analysis into your CRM ensures that all teams–sales, marketing, and customer support–have access to meaningful insights, facilitating proactive decision-making.
Steps to Integration
- Assess the current CRM capabilities and identify gaps in sentiment analysis functionalities.
- Choose a knowledge-based sentiment analysis tool that supports the cryptocurrency domain.
- Integrate the tool via API or direct integration, ensuring that all relevant data sources are connected (social media feeds, news platforms, etc.).
- Train your CRM system to recognize cryptocurrency-specific jargon and slang to enhance accuracy.
- Monitor and adjust sentiment models regularly to reflect changes in market behavior and trends.
Benefits of Knowledge-Based Sentiment Integration
- Improved Customer Interaction: Sentiment analysis helps anticipate customer needs and adjust messaging accordingly, improving customer service.
- Real-Time Market Insights: Understand fluctuations in public sentiment about cryptocurrencies to adjust your offerings and marketing strategies quickly.
- Personalized Recommendations: Use sentiment data to provide personalized product recommendations based on a customer’s emotional response to particular cryptocurrencies.
Tip: Regularly update your knowledge base to account for the dynamic nature of cryptocurrency trends, ensuring your sentiment analysis stays relevant and accurate.
Example of Sentiment Data Integration
Cryptocurrency | Sentiment Score | Customer Feedback |
---|---|---|
Bitcoin | Positive (78%) | Customers are optimistic about long-term stability and trust in Bitcoin. |
Ethereum | Neutral (50%) | Mixed reviews, with some concerns over scalability and gas fees. |
Ripple | Negative (35%) | Customer sentiment is largely negative due to legal battles and regulatory issues. |
Key Advantages of Knowledge-Driven Sentiment Analysis in Crypto Market Research
Knowledge-based sentiment analysis is gaining increasing importance in the cryptocurrency market, where volatility and rapid price fluctuations make real-time data interpretation crucial. This approach goes beyond simple emotion detection by integrating domain-specific expertise and insights into the analysis, leading to more accurate sentiment assessments. By leveraging historical data, blockchain activity, and market indicators, knowledge-driven sentiment analysis provides a nuanced understanding of market psychology, allowing investors to make informed decisions.
For crypto market research, applying sentiment analysis that is informed by expert knowledge brings several advantages. It can help identify trends before they manifest in price movements, giving traders an edge in predicting market shifts. Moreover, it provides more granularity in understanding the sentiments surrounding specific tokens, influencers, or events, creating a deeper insight into the factors that drive price changes. Below are key benefits this approach offers:
Key Benefits
- Enhanced Accuracy: By incorporating industry-specific knowledge, the analysis captures complex market sentiment more accurately than traditional methods.
- Early Trend Detection: Knowledge-based models help detect emerging trends faster, giving traders a competitive advantage in identifying potential market shifts.
- Deep Insights into Influences: Sentiment analysis informed by market knowledge can distinguish between short-term noise and long-term influences, providing a clearer picture of the forces at play.
Important Considerations:
Integrating expert knowledge into sentiment analysis models requires constant updates and validation to stay relevant in the fast-paced cryptocurrency market.
Comparison of Sentiment Analysis Approaches
Method | Pros | Cons |
---|---|---|
Traditional Sentiment Analysis | Fast, broad coverage, simple implementation | Lacks depth, less accurate for crypto market specifics |
Knowledge-Based Sentiment Analysis | More precise, considers expert input and crypto-specific data | Requires ongoing updates, more complex implementation |
Understanding the Role of NLP in Knowledge-Driven Sentiment Analysis in Cryptocurrency
Natural Language Processing (NLP) has become an essential tool for analyzing sentiment in the cryptocurrency market, where the perception of investors and users can significantly influence market behavior. By processing vast amounts of textual data, such as news articles, social media posts, and forum discussions, NLP techniques allow for the extraction of insights that help identify positive, negative, or neutral sentiments towards cryptocurrencies. This is crucial for predicting market trends and understanding investor sentiment, which often drives price fluctuations in this volatile market.
In knowledge-driven sentiment analysis, NLP leverages existing domain-specific knowledge and integrates it with linguistic models to create more accurate interpretations of sentiment. By understanding the terminology, slang, and context within the cryptocurrency world, NLP systems can process user-generated content more effectively. This allows stakeholders, such as traders and analysts, to make informed decisions based on real-time sentiment trends.
Key Steps in Knowledge-Based Sentiment Analysis for Cryptocurrency
- Data Collection: Gathering data from diverse sources like social media, cryptocurrency forums, news platforms, and blogs.
- Text Preprocessing: Cleaning and structuring the data to make it usable for sentiment analysis algorithms.
- Sentiment Classification: Using NLP techniques to classify text into positive, negative, or neutral categories.
- Knowledge Integration: Incorporating domain knowledge such as common cryptocurrency terminology and market events to improve analysis accuracy.
- Real-time Analysis: Continuously analyzing incoming data to track fluctuations in sentiment and adjust predictions accordingly.
Example of Sentiment Analysis Results for Cryptocurrency Tweets
Sentiment | Example Tweet | Score |
---|---|---|
Positive | "Bitcoin's recent surge is incredible! I think it's heading to the moon!" | +0.85 |
Negative | "Ethereum's price drop is worrying. It might be a sign of a bigger crash." | -0.72 |
Neutral | "Bitcoin's market cap is over $1 trillion now. Let's see where it goes from here." | 0.05 |
Important Note: Knowledge-based sentiment analysis benefits from incorporating both general sentiment models and cryptocurrency-specific knowledge to improve accuracy and relevance. This approach is more efficient than relying solely on traditional sentiment analysis techniques.
How to Train Your Sentiment Analysis Model for Accurate Results in Cryptocurrency
Training a sentiment analysis model for cryptocurrency markets requires a specialized approach, given the volatility and rapid changes in sentiment. Unlike traditional industries, cryptocurrency discussions are highly influenced by social media trends, news events, and market speculation. To achieve accurate predictions, it is essential to focus on the specific language, emotions, and patterns that influence market behavior in the crypto space.
Effective sentiment analysis training involves three main components: data acquisition, feature selection, and model evaluation. The goal is to identify the sentiment driving market fluctuations, whether positive, negative, or neutral, and tailor your model to recognize those signals. Below are the essential steps to create a reliable sentiment analysis model for cryptocurrency.
Key Steps for Training Your Sentiment Model
- Data Collection: Gather cryptocurrency-specific data from sources like Twitter, Reddit, and news articles. Make sure to include various market conditions, including price fluctuations, ICO announcements, and regulatory changes.
- Data Preprocessing: Clean the collected data by removing stopwords, special characters, and irrelevant content. Tokenization and stemming are crucial to normalize text.
- Labeling Data: Manually label a sample of the data or use semi-supervised learning to label data based on market outcomes (e.g., price increase = positive sentiment, decrease = negative sentiment).
- Feature Extraction: Use natural language processing (NLP) techniques like TF-IDF, word embeddings, and sentiment lexicons tailored for cryptocurrency terms (e.g., "bullish," "bear market," "HODL").
Evaluation & Fine-Tuning
Once your model is trained, fine-tuning is necessary to ensure high accuracy in real-world scenarios. Evaluate the model's performance with metrics such as precision, recall, and F1-score. A common issue is the imbalance in data–positive news often outweighs negative news, which can skew results. To counteract this, use techniques like oversampling, undersampling, or class weighting during training.
"A good sentiment analysis model will not only classify the sentiment but will also adapt to the evolving language used within the cryptocurrency community. The speed at which language evolves in the crypto world is a challenge, but continuous retraining and tuning can address this."
Sentiment Model Evaluation Table
Metric | Description | Importance |
---|---|---|
Precision | Measures the proportion of true positive results out of all predicted positives. | High precision is critical for ensuring that the model doesn’t classify neutral or negative sentiment as positive. |
Recall | Measures the proportion of true positive results out of all actual positives. | Recall is important for capturing all relevant positive sentiments that could influence market movements. |
F1-Score | Harmonic mean of precision and recall, balancing both metrics. | F1-Score is useful for balancing both false positives and false negatives in market sentiment prediction. |
Real-Time Sentiment Monitoring in Cryptocurrency Markets
In the fast-paced world of cryptocurrency trading, capturing sentiment in real time is essential for understanding market dynamics. Traders, investors, and analysts rely heavily on sentiment analysis to make informed decisions, as emotions and public opinions can drastically influence the value of digital assets. With constant news cycles, social media buzz, and forum discussions, real-time sentiment analysis provides a critical edge in predicting price movements and identifying trends as they emerge.
Sentiment analysis in cryptocurrency markets focuses on gathering and interpreting data from multiple sources, including social media platforms, news outlets, and market forums. The real-time aspect is crucial because trends can shift rapidly, often within hours or even minutes. Understanding how to capture and analyze this sentiment accurately allows market participants to react quickly and make decisions based on the latest public sentiment rather than relying solely on traditional indicators.
Key Approaches to Real-Time Sentiment Tracking
- Social Media Monitoring: Platforms like Twitter, Reddit, and Telegram are rich sources of real-time sentiment data. Analyzing the frequency and tone of posts can reveal market sentiment shifts.
- News Sentiment Analysis: Monitoring news articles for sentiment allows traders to respond to breaking news events that could affect cryptocurrency prices.
- Market Sentiment Analysis: Examining price movement alongside social media sentiment can help identify correlations and predict future trends.
Steps for Capturing Trends
- Data Collection: Gather real-time data from various online platforms and news sources.
- Sentiment Classification: Use natural language processing (NLP) algorithms to classify posts or articles as positive, negative, or neutral.
- Trend Identification: Identify patterns in sentiment over time and correlate them with market movements.
- Actionable Insights: Provide recommendations based on the sentiment trends, helping traders make timely decisions.
Real-time sentiment analysis is an invaluable tool for those involved in cryptocurrency markets, where prices can fluctuate rapidly based on public perception.
Sample Sentiment Data Tracking Table
Platform | Sentiment Score | Trending Asset | Timestamp |
---|---|---|---|
+0.75 | Bitcoin | 2025-03-23 10:30 | |
-0.45 | Ethereum | 2025-03-23 11:00 |
Overcoming Common Challenges in Knowledge-Based Sentiment Analysis Implementation
In the domain of cryptocurrencies, implementing knowledge-based sentiment analysis faces several unique challenges. These obstacles primarily stem from the highly dynamic and decentralized nature of the market, as well as the continuous influx of user-generated content across various platforms. Accurate sentiment extraction becomes increasingly difficult as the tone of discussions fluctuates rapidly, and interpreting nuanced expressions in this volatile environment is far from straightforward. Furthermore, limited availability of reliable structured data and specialized language models for cryptocurrency-related content only compounds the difficulty of the analysis.
Another key difficulty in the knowledge-based sentiment analysis process is dealing with the ambiguous context surrounding certain phrases or slang commonly used within cryptocurrency communities. Identifying whether a term has a positive, negative, or neutral implication can be highly dependent on the context in which it is used. This issue becomes even more complex when accounting for factors such as regional dialects and variations in online jargon, which makes training sentiment analysis models challenging.
Challenges in Knowledge-Based Sentiment Analysis
- Context Ambiguity: The use of slang, abbreviations, and context-dependent terms in cryptocurrency discussions can skew sentiment analysis.
- Dynamic Market Changes: Cryptocurrency market sentiment changes rapidly, requiring adaptive models to stay relevant.
- Data Scarcity: Lack of structured and labeled data specific to the cryptocurrency domain limits the accuracy of sentiment analysis models.
Solutions and Strategies for Improvement
- Advanced Contextual Models: Leveraging models such as BERT (Bidirectional Encoder Representations from Transformers) can significantly enhance the ability to interpret the context of phrases, reducing ambiguity in sentiment analysis.
- Continuous Data Collection: Using real-time data from social media, news, and forums allows the model to adapt to rapidly changing market trends and sentiment.
- Building Domain-Specific Lexicons: Constructing a specialized lexicon focused on cryptocurrency-related terms helps in better handling industry-specific terminology and sentiment nuances.
"Cryptocurrency markets are inherently volatile, and this volatility influences public sentiment in ways that traditional sentiment analysis models often fail to capture. Adapting to these shifts requires continuous model training and refinement."
Example of Knowledge-Based Sentiment Analysis in Cryptocurrencies
Phrase | Sentiment |
---|---|
Bitcoin hitting new highs | Positive |
Ethereum experiencing major drops | Negative |
Ripple facing regulatory issues | Negative |